Credit: Kanur Ismail / 3d_kot via iStock
Technology has made it possible for companies to measure just about everything — from in-person behaviors to remote sensor activity — in the hopes of making better, quicker decisions. But true progress involves a lot more than simply collecting information.
Companies need to remember that measurement isn’t the same as insight, said Joel Shapiro, a professor at Northwestern University’s Kellogg School of Management, during a recent MIT Sloan Management Review webinar.
“One of the most important things that you can do to be successful with data is to reframe data as evidence,” said Shapiro, who leads Kellogg’s Analytical Consulting Lab. “Just because we measure things doesn’t mean we necessarily know what to do about [them].”
Unlike data, which consists of information like numbers or words, evidence is contextual and directional. “When we start talking about evidence instead of data, that’s when we start talking about important things, like ‘What is the problem that we’re trying to solve?’” Shapiro said.
During his presentation, titled “Making Data Indispensable: Frameworks for Effective Data Leadership,” Shapiro offered insight on how to get the most business value from data. Here are four tips to help leaders use their data for maximum impact.
1. Modernize your data technology.
As companies implement generative artificial intelligence in the workplace, they need to consider whether they should improve or replace their data technology, too. Thinking about how you store data and how transparent you are about how it’s used can also help in identifying and mitigating ethical risk.
“About 10 or 12 years ago, when data first got really hot, we saw teams building some solid technology, and they sort of kept pace for a little bit,” Shapiro said. However, “within the last couple of years as generative AI has gotten really hot and really important, teams have realized that they need to improve their data.”
Shapiro suggested four questions that companies should ask when trying to determine how reliable and capable their technology is.
- Can we get the information we need quickly?
- Do we have adequate speed, scalability, and flexibility?
- Can we handle growth without a lot of manual or ad hoc workarounds?
- Can we integrate new data sources without complexity or delay?
2. Match your business need to your data capabilities.
Shapiro said that companies must use data that is appropriate for their business objective.
For example, if someone were in charge of analytics and data science for building an e-commerce platform, they’d want to do A/B testing and engage in causal inference to gain an understanding of whether the layout on the page worked — tasks that revolve around experimentation, for the most part, Shapiro said.
On the other hand, if someone wanted to build a predictive model in an oncology unit, they might want to use machine learning and look at a lot of images to gain a better understanding of what patterns might be predictive of cancer, which is “very, very different than running experiments in causal inference,” he said.
Both of these examples “are very different from a company that just wants to build dashboards and monitor something like cash flow or cash burn on a weekly basis,” Shapiro said. “These are important elements of quality of data beyond just ‘Is it clean?’”
In addition to ensuring data quality, data leaders should identify and align on key metrics and visualize data in ways that resonate with the C-suite, to ensure that executives trust the data.
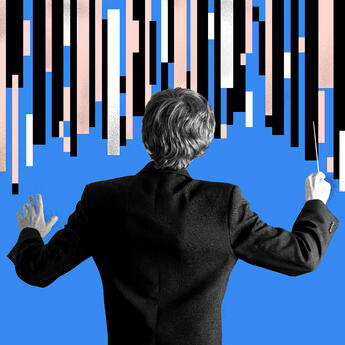
Leading the AI-Driven Organization
In person at MIT Sloan
Register Now
3. Measure the return on your investment.
Shapiro said that companies have been doing a good job of uncovering their business problems and building out data capabilities to solve them, but they still “need to invest where data actually moves the needle, and they must measure return on investment on that relentlessly.”
When they do, they get better at investing in the right places, Shapiro said. Pilot tests and historical simulations are great places to start.
If buy-in from management remains an obstacle, “try your best to measure return on investment as quickly as possible,” Shapiro said. “I think the best way to approach it is to tie it to a real and material business problem that you’re actually facing.”
4. Make sure your organization knows how to use the output — and is committed to taking action.
It’s crucial that companies know how to use the output of their findings. Shapiro recommended that companies think about the following when implementing their plans.
- Train employees on how to use data. Otherwise, “it’s going to be really, really hard to be successful with data,” Shapiro said.
- If a model suggests change, stay open to taking the recommended course of action. “If you’ve got a model or data telling you that you have to totally reorganize your entire department or completely change up the ways in which you work, that can be a little bit daunting for people,” Shapiro said. Data-driven companies stay the course and actually make those changes.
- Incentivize employees to use data. “This is where a lot of companies fall down, and I kind of am surprised by it,” Shapiro said. Fostering a culture of curiosity and accountability can go a long way toward building high-performing data teams.
- Beef up your communication skills when talking about data. “Do you have clarity, and do you have purpose?” Shapiro asked. You need to be “really, really cognizant” of what you’re trying to say.
- “Make sure you’ve got a well-articulated problem; that you’ve got the right capability to match up with that, whether it’s experiments or machine learning predictions or dashboards; and [that you’re] measuring the ROI — those are the most important things,” Shapiro said.
Read next: 3 ways to build a culture of data monetization